Image Generated Using DALL-E
An AI Accelerator is specialized hardware designed to process artificial intelligence (AI) tasks efficiently. These accelerators are specifically optimized to handle computations related to machine learning and deep learning algorithms, which are the core of most modern AI applications.
Key features and purposes of AI Accelerators include:
Efficiency: Designed to be more efficient than general-purpose processors like CPUs (Central Processing Units) for AI tasks. This efficiency comes from faster processing times due to higher throughput via multiple processing elements.
Parallel Processing: AI algorithms benefit from similar processing capabilities, especially those in neural networks. AI accelerators often have architectures that support high degrees of parallelism, allowing them to process multiple operations simultaneously.
Optimized Operations: AI accelerators get optimized for the types of mathematical operations most common in AI workloads, such as matrix multiplication and vector operations, which are crucial for neural network computations.
Memory Bandwidth: High memory bandwidth is essential for AI workloads, and these accelerators often have specialized memory architectures to support fast data access.
Scalability: AI accelerators can be scaled to support larger models and datasets, which is vital as the complexity of AI tasks continues to grow.
These features make AI accelerators indispensable in various applications, ranging from natural language processing and image recognition to autonomous vehicles and advanced analytics, driving innovation and efficiency in AI development and deployment.
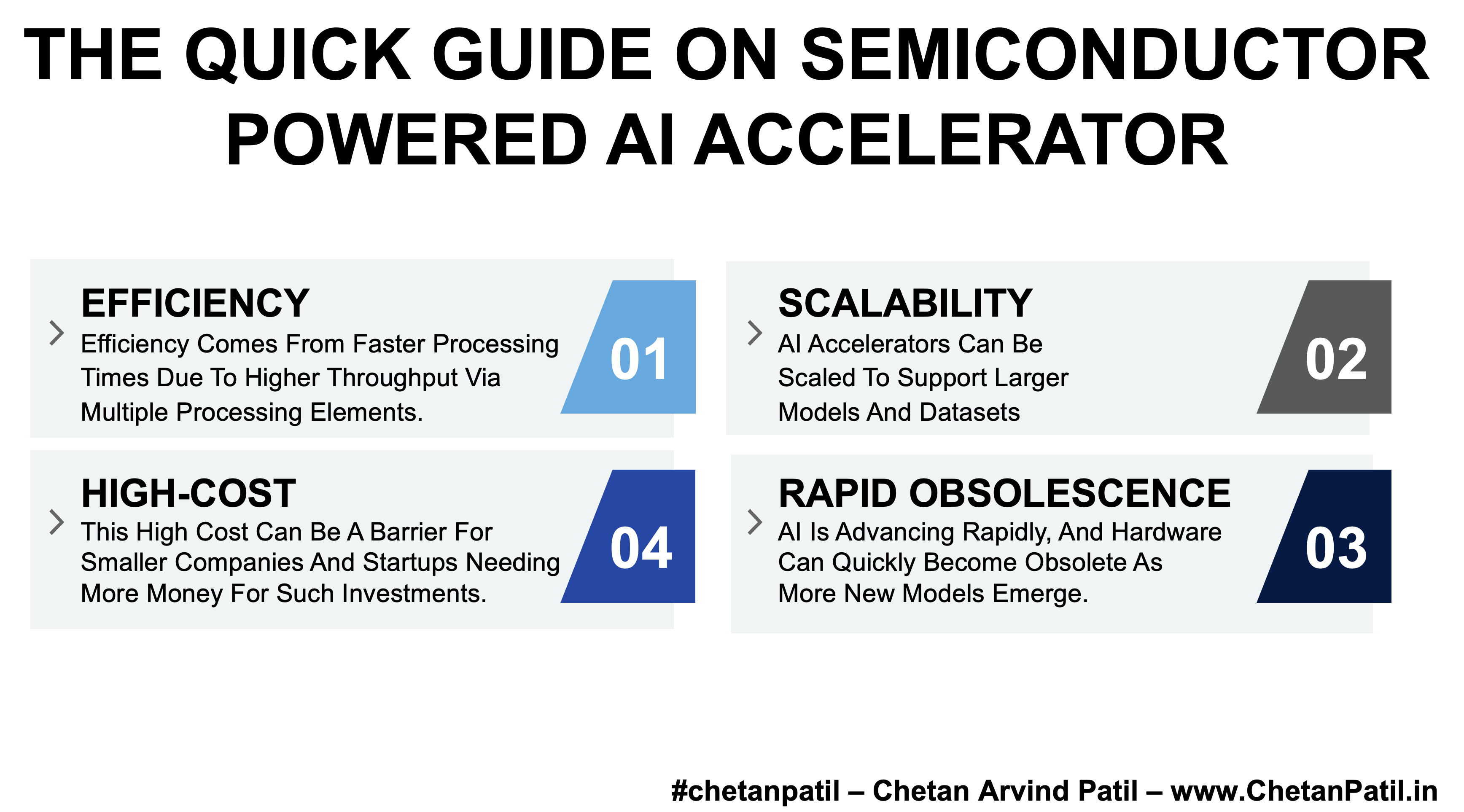
AI accelerators, despite their numerous advantages in enhancing the performance of AI and machine learning tasks, also come with certain drawbacks:
High Cost: AI accelerators, especially the more advanced models, can be expensive. This high cost can be a barrier for smaller companies and startups needing more money for such investments.
Specialized Hardware Requirements: Since these accelerators are specialized hardware, integrating them into existing systems can sometimes be challenging. They may require specific motherboards, power supplies, and cooling systems, which adds to the complexity and cost.
Limited Flexibility: Some AI accelerators, particularly ASICs like TPUs, are highly optimized for specific tasks or computations. This specialization can limit their flexibility, making them less suitable for a broader range of applications or emerging AI algorithms requiring different computational capabilities.
Software Ecosystem And Compatibility: AI accelerators rely heavily on software and frameworks compatible with their architecture. This dependency means that changes or updates in software could necessitate adjustments in the hardware or vice versa, potentially leading to compatibility issues.
Complexity In Programming And Maintenance: Programming AI accelerators requires specialized knowledge and skills, particularly for optimizing the performance of AI models. Additionally, maintaining these systems, both in terms of software and hardware, can be complex and resource-intensive.
Power Consumption And Heat Generation: High-performance AI accelerators can consume significant power and generate considerable heat, especially in large data centers. It necessitates sophisticated cooling solutions and can lead to higher operational costs.
Scalability Challenges: While AI accelerators are scalable, scaling them to extensive systems can be challenging and expensive, especially in data center environments where thousands of accelerators might be required, leading to increased complexity in infrastructure, power, and cooling requirements.
Rapid Obsolescence: AI and machine learning are advancing rapidly, and hardware can quickly become obsolete as more new models emerge. This fast pace of development can make it challenging for organizations to keep up with the latest technology without significant ongoing investment.
In conclusion, AI accelerators significantly advance artificial intelligence and machine learning, offering unparalleled efficiency and performance for complex computational tasks. These specialized hardware components have become crucial in powering a wide range of AI applications, from deep learning models in data centers to real-time processing in edge devices.
While they offer substantial benefits regarding processing speed and energy efficiency, challenges, such as high cost, specialized hardware requirements, limited flexibility, and rapid obsolescence, must be carefully considered. As the AI landscape continues to evolve rapidly, AI accelerators stand as a testament to the ongoing synergy between hardware innovation and software development, driving forward the capabilities and applications of AI technology in an increasingly digital world.